Evolution of Game Theory in CSGO
Over the years, Counter-Strike has evolved from a basic first-person shooter into a complex battlefield where game theory plays a pivotal role. Strategic thinking in Counter-Strike involves predicting opponents' moves and counterstrategies. By 2024, these strategies had grown even more nuanced with the introduction of new game mechanics and features.
Game theory within Counter-Strike focused largely on risk-reward decisions, such as purchasing weaponry that could dictate early game momentum or saving funds for later rounds. This layer of strategic depth added a psychological element to gameplay.
The introduction of new maps and tweaks in weapon balance further shifted how strategies were formulated. Each map alteration required teams to adapt continually or face being outmaneuvered.
The addition of the 'Fog of War' feature, which limits a player's vision to line-of-sight, demanded new strategies. Players needed heightened communication and had to predict enemy movements without direct visual confirmation.
Team dynamics and roles have become more sophisticated. By 2024, roles had become more defined, transforming team strategies into an interplay of specialized roles working together according to predefined strategies and contingencies.
Economic strategies in Counter-Strike grew increasingly complex. Decisions regarding the team's economy—like whether to buy, save, or attempt a 'force-buy' when funds are low—embraced a deeper understanding of probability and risk management.
The impact of artificial intelligence by 2024 provided new data-driven approaches to training and strategy formulation. Teams began leveraging AI tools to analyze gameplay data to uncover trends and potentially predict opponents' behaviors.
As Counter-Strike continues to evolve, the application of game theory moves from strength to strength, mimicking real-world strategies and decision-making conflicts. Each strategic layer contributes to the gameplay that both challenges and rewards strategic thinkers.
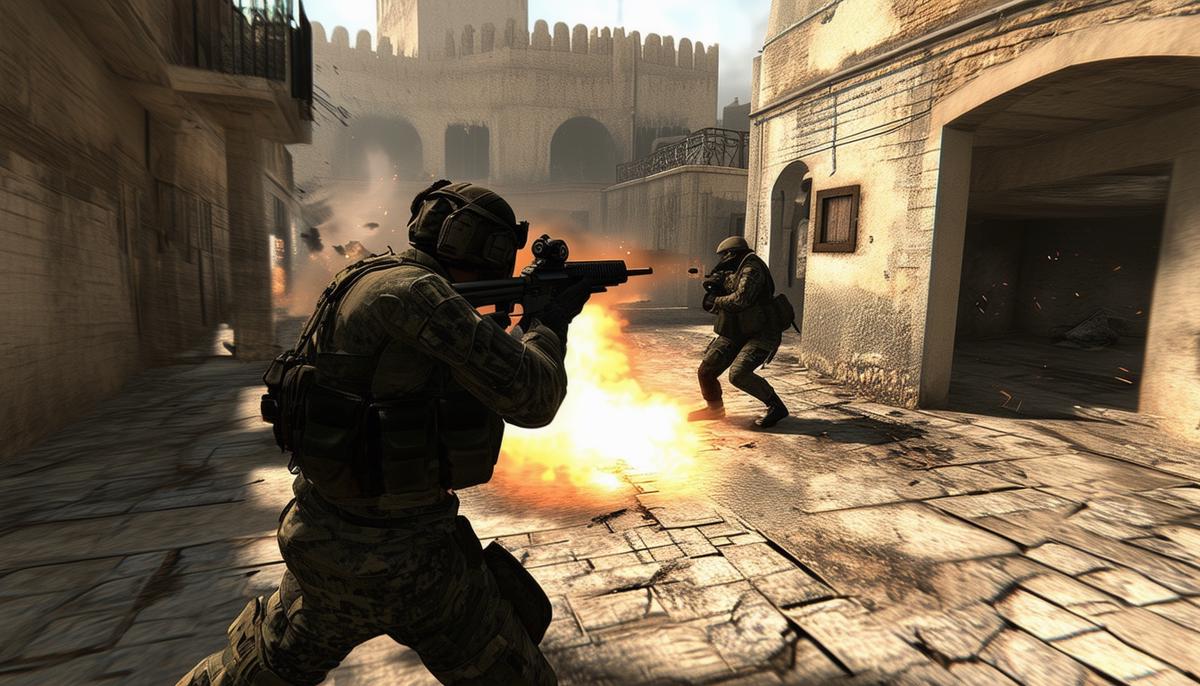
Current Strategies and Meta
In the present strategic landscape of CSGO, the meta revolves around leveraging map control and timing pushes while counter-strategizing through utility usage like smoke bombs and flashbangs to disrupt enemy lines. The complexity of game theory is exemplified during post-plant situations, where teams shift their focus toward delay tactics or retrieving lost control.
Top players and teams deeply integrate game theory into their play styles. A prevalent tactic involves economic manipulation where teams intentionally lose specific rounds to break the opponent's monetary cycle. Economically starving out opponents causes them to have reduced utility and inferior weaponry, which can be exploited strategically.
Strategic depth in gameplay is further seen in choice deployment of utility. Top-tier players calculate the perceived value of using utilities in different scenarios.
Team compositions pivot on role specification. A 'lurker' can apply pressure by engaging independent from their team to create opportunities. Designated role players like 'entry fraggers' and 'awpers' emphasize precision and timing in their movements syncing with supportive teammates.
Analysis of map-specific strategies underscores the sophistication of current gameplay. Control over key areas can provide essential flexibility for both attacking and defending teams, often dictated by sniper duels.
The increasing application of artificial intelligence and analytics tools also fuels the complexity of current strategies. Teams with access to game data can perform deeper analysis on map sequences, smoke setups, or player positioning patterns.
As the boundary between technology-driven insight and human tactical intuition blends, teams that excel are those capable of effortless synergy between these components. Players' adaptability to rapid meta shifts, understanding of opponent strategy tendencies, and capability for real-time critical analysis amidst high-stress moments are the benchmarks distinguishing top league participants.
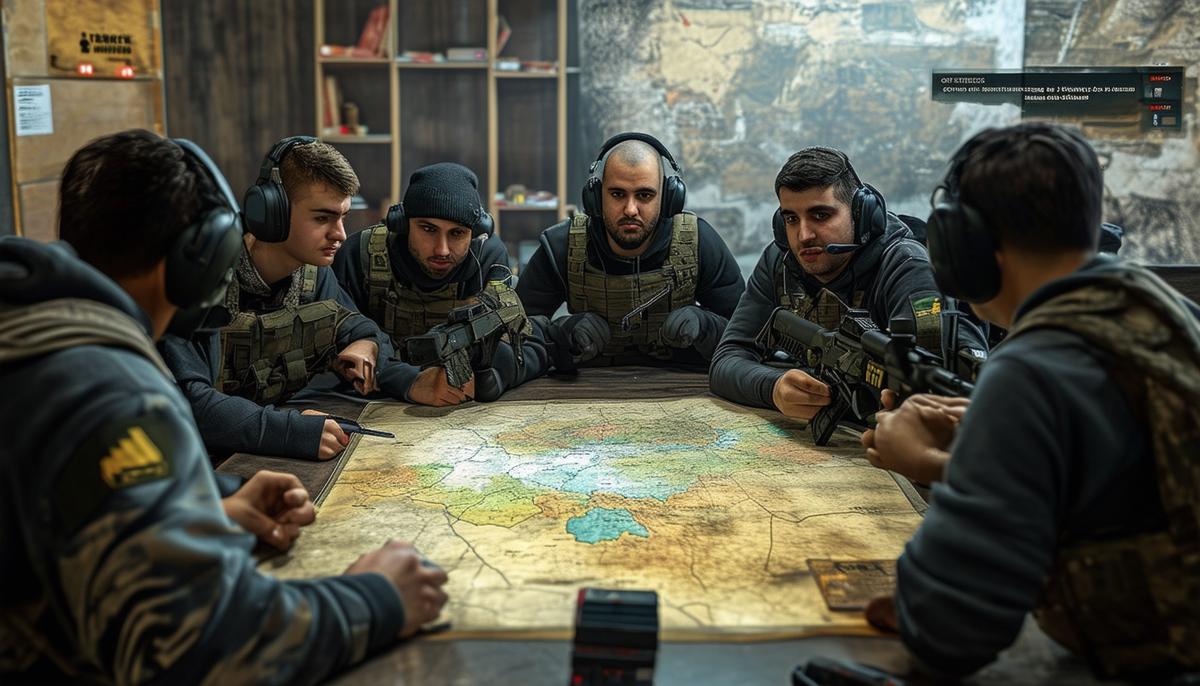
Impact of AI and Machine Learning
The integration of AI and machine learning technologies into 'Counter-Strike: Global Offensive' significantly reshapes how strategies are devised and executed. The impact of these advancements influences various aspects of gameplay, from individual training routines to team-wide strategy formulation and real-time decision-making.
AI algorithms analyze gameplay data extensively. Teams harness these tools to dissect countless hours of match footage to identify underlying patterns in enemy moves or potential weak spots in map control. Such analytics enable teams to plan their game strategies, predicting opponents' tactics with greater accuracy.
The depth of strategic insights gleaned from AI extends into personalized player training. Custom training modules generated from AI analysis help players focus on specific areas of improvement.
The utilization of machine learning streamlined the assimilation and adaptation process to rapidly changing game metas. AI can simulate millions of potential strategies offering insights into the most effective tactics under present competitive conditions.
During matches, real-time decision-making is augmented by AI-powered tools that offer instantaneous recommendations based on live gameplay. AI interfaces provide valuable tactical support on the fly through rapid assessment of ongoing game dynamics and historical performance patterns.
The incorporation of AI promises more democratically accessible improvement opportunities across varying skill levels. Smaller teams or amateur players, equipped with AI tools, can level the competition against more established teams by accessing sophisticated strategic analysis.
While the integration of AI and machine learning offers immense advantages, it also poses new challenges around adaptation and continuous learning as human elements interface more profoundly with technological advancements. The future competitive arena in Counter-Strike might be a testament to superior tactical knowledge and reflexes and a showcase of how well players and teams can fuse cognitive prowess with cutting-edge technology to craft winning strategies.
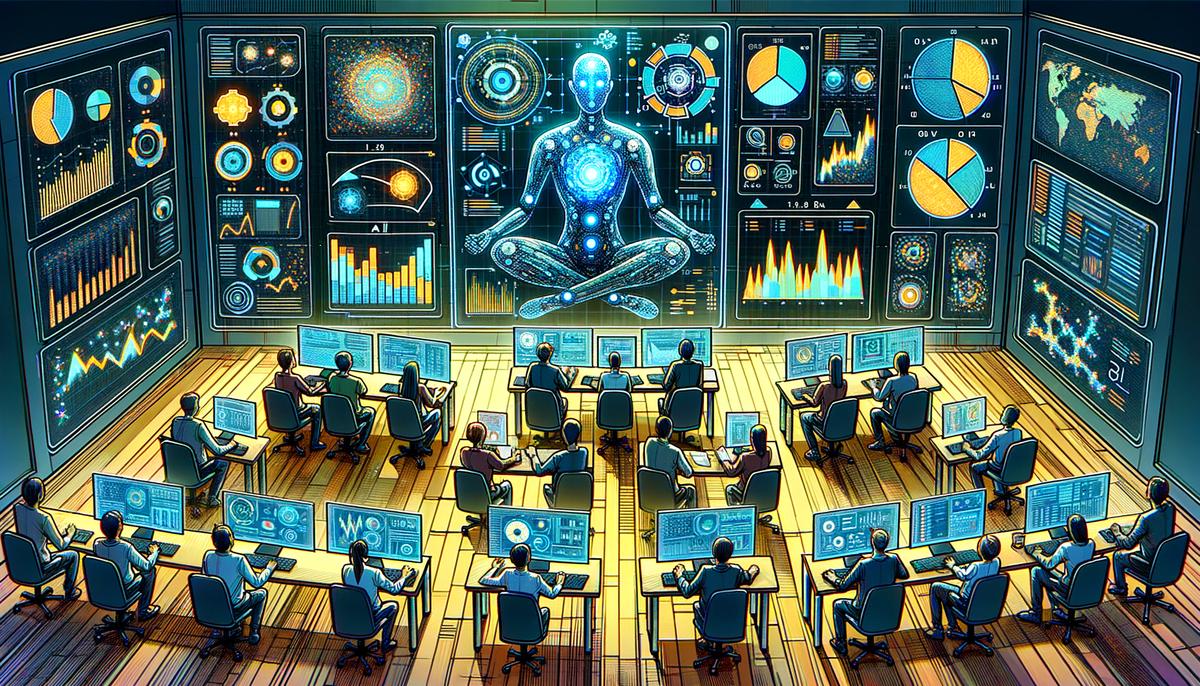
Future Predictions and Trends
Future trends in game theory within 'CSGO' are expected to intersect significantly with continuous technological advancements. As tools and analytics systems become more sophisticated, the implementation of virtual reality (VR) and augmented reality (AR) could fundamentally transform training methodologies and in-game dynamics.
The potential integration of VR could bring about a holistically immersive environment where players can engage in near-real conditions, thereby practicing tactics and team strategies with a realism level far beyond current capabilities. VR technology could enable players to study and familiarize themselves with game terrains in a full-scale manner.
AR could provide real-time data overlays during matches, offering players statistics like trajectory angles or sniper line sights that would refine tactical decisions under fast-paced circumstances. This inclusion of AR might evolve the in-game experience to more closely resemble a high-tech battleground where strategic moves are informed by immediate data insights.
The potential evolution of game rules, possibly influenced by both player input and spectator engagement demands, could introduce fresh tactical challenges and revitalization of game theory elements, as teams strategize within a transformed rule set.
Player skills are anticipated to become more diverse with an increased emphasis on multidisciplinary abilities. Future professional players might need to evolve beyond strategic foresight or tactical knowledge, stepping into realms of rapid adaptation to new technologies or proficiency in utilizing real-time data during gameplay.
The growing shift towards AI and machine learning might push towards even more personalized gameplay adjustments. As AI becomes more adept at predicting individual player behaviors and tendencies, game theory could become hyper-personalized.
In academia, the advancement in understanding human decision-making and game theory potentiated by esports analytics could break new ground in cognitive science and applied mathematics. The intersection of game design, computational data analysis, and psychological assessment might lead to burgeoning new research fields and educational curriculums focusing on strategic thinking and decision intelligence.
Amidst these technological and rule-evolving changes, the cerebral aspect of 'Counter-Strike: Global Offensive' will continue to be its cornerstone. 'CSGO' solidifies itself as a herald of esports evolution and a vibrant arena for showcasing the power of human strategy when it converges with the technological forefront.
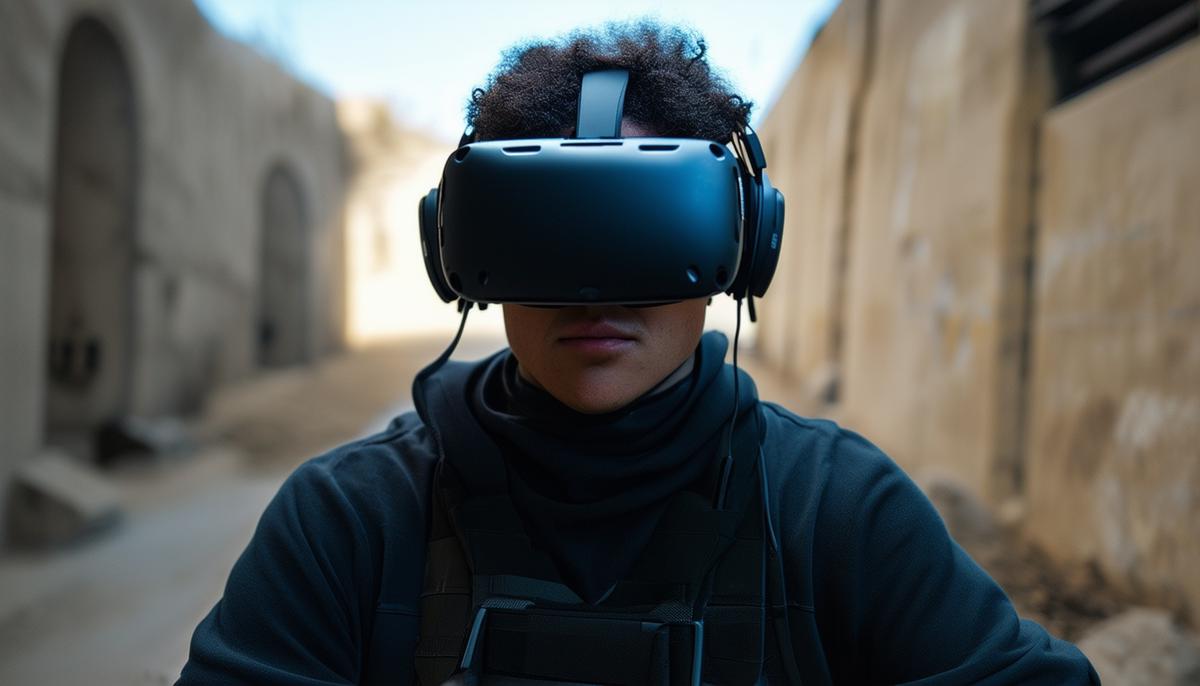
Unleash the power of AI with Writio! Your content, our magic. This article was written by Writio.
- Agarwal S, Deepmind A. Counterfactual regret minimization: a reinforcement learning perspective. J Artif Intell Res. 2021;71:287-317.
- Burch N, Schmid M, Moravcik M, Morill D, Bowling M. Aivat: a new variance reduction technique for agent evaluation in imperfect information games. In: Proceedings of the AAAI Conference on Artificial Intelligence. Vol 32. No 1. 2018.
- Esche M, Kluss T, Tuyls K, Neumann A. A theoretical and empirical analysis of variance reduction for policy gradient methods. arXiv preprint arXiv:2107.02036. 2021 Jul 5.
- Ye D, Chen G, Zhang W, Chen S, Yuan B, Liu B, Chen J, Liu Z, Qiu F, Yu H, Yin Y. Towards playing full MOBA games with deep reinforcement learning. Adv Neural Inf Process Syst. 2020;33:621-32.
Leave a Reply